Applying sensing and AI to innovative problem solving
Sprawling municipalities face many challenges. Traffic congestion slows productivity, contributes to air quality problems, and wastes energy. Crowds of people bring concerns over public health, safety, and security. Distributed sensors help monitor conditions, but in turn generate volumes of big data. AI inference helps cut through routine monitoring, checking compliance, spotting problems, or highlighting opportunities for savings.
Urban environments can be a great setting for people watching, but in some cases a more quantitative approach is needed. Smart surveillance can apply to any gathering place in a city - rooms in a conference center, streets and walkways, and special events.
Networks of cameras provide high-resolution video from many angles, and volumes of data. The task of watching all that data would numb human operators, opening the chance that something important is missed. AI inference is proving valuable in accurate recognition of people and items they wear or carry.
Simple AI inference models are now highly accurate at smart surveillance. Tasks like verifying room capacity, checking mask compliance, and assessing foot traffic can be automated, allowing human resources to be deployed for other priorities.
Traffic is a concern as cities grow and freeways and thoroughfares become more congested. A side effect of increased traffic is difficulty finding parking. Helping traffic flow more efficiently is a good application for AI inference in spotting patterns.
Traffic congestion also impacts the perceived livability of a city. It increases commute times, drives up fuel consumption, adds to air pollution, and frustrates drivers. Building more roads or adding lanes may alleviate problems in one area, but the point of congestion usually moves to another spot. More timely information is needed.
Traffic management systems gather information from many sources - like cameras, in-road sensors, vehicle-to-everything (V2X) systems - creating a better picture of what is happening in real-time. With that data, signals can be controlled, signage can indicate alternate routes, tolls can be automated, keeping vehicles moving.
Retailers are always looking for ways to enhance the experience of their shoppers, and increase revenue per visit. Smart retail systems with AI inference help match shoppers to goods and entice them with offers tailored for their preferences.
Smart retail systems can help guide shoppers from the moment they enter the store to items of interest. Along the way, shoppers might pass digital signage displays with cameras gathering more non-personally identifiable information - gender, age range, clothing and accessories. With that information, dynamic offers can be presented.
Smart retail may also work with other sensors, like RFID-enabled shopping carts that sense what items a shopper has selected, making complementary suggestions. Traffic flow and signage watch time are also important metrics for optimizing placements.
SAKURA-II M.2 Modules and PCIe Cards
EdgeCortix SAKURA-II can be easily integrated into a host system for software development and AI model inference tasks.
Order an M.2 Module or a PCIe Card and get started today!
EdgeCortix Edge AI Platform
Full-Stack Solution: Integrated Platform Increases Ecosystem Value Over Time
Unique Software
Proprietary Architecture
AI Accelerator
Efficient Hardware
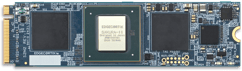
Modules and Cards
powered by the latest SAKURA-II
AI Accelerators
Deployable Systems
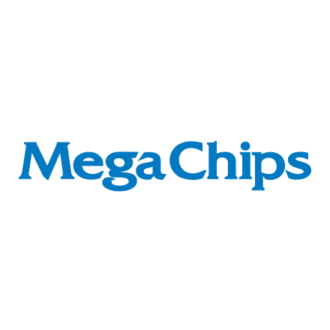
Given the tectonic shift in information processing at the edge, companies are now seeking near cloud level performance where data curation and AI driven decision making can happen together. Due to this shift, the market opportunity for the EdgeCortix solutions set is massive, driven by the practical business need across multiple sectors which require both low power and cost-efficient intelligent solutions. Given the exponential global growth in both data and devices, I am eager to support EdgeCortix in their endeavor to transform the edge AI market with an industry-leading IP portfolio that can deliver performance with orders of magnitude better energy efficiency and a lower total cost of ownership than existing solutions."
Improving the performance and the energy efficiency of our network infrastructure is a major challenge for the future. Our expectation of EdgeCortix is to be a partner who can provide both the IP and expertise that is needed to tackle these challenges simultaneously."
With the unprecedented growth of AI/Machine learning workloads across industries, the solution we're delivering with leading IP provider EdgeCortix complements BittWare's Intel Agilex FPGA-based product portfolio. Our customers have been searching for this level of AI inferencing solution to increase performance while lowering risk and cost across a multitude of business needs both today and in the future."
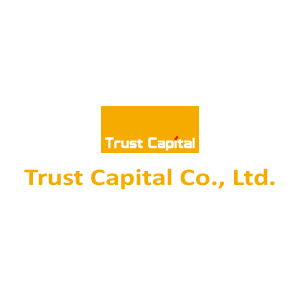
EdgeCortix is in a truly unique market position. Beyond simply taking advantage of the massive need and growth opportunity in leveraging AI across many business key sectors, it’s the business strategy with respect to how they develop their solutions for their go-to-market that will be the great differentiator. In my experience, most technology companies focus very myopically, on delivering great code or perhaps semiconductor design. EdgeCortix’s secret sauce is in how they’ve co-developed their IP, applying equal importance to both the software IP and the chip design, creating a symbiotic software-centric hardware ecosystem, this sets EdgeCortix apart in the marketplace.”